- Share
- Share on Facebook
- Share on X
- Share on LinkedIn
Séminaire
On February 20, 2023
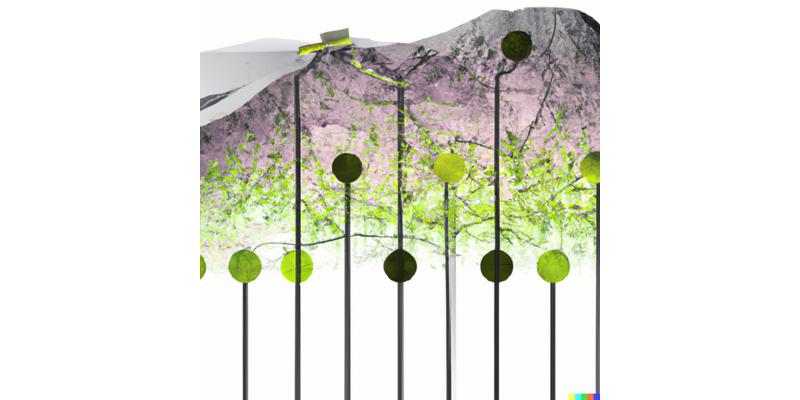
Victor Boussange (ETH Zürich)
In the recent years, the field of artificial intelligence has made enormous progresses in computer vision and natural language processing. At the backbone of this success are data-driven models that can learn abstract representation of mechanisms from large datasets. Yet, these abstractions can hardly be interpreted to generate scientific knowledge, and the success of these methods is greatly limited by the information contained in the datasets. Nevertheless, the key computational techniques they rely upon could be blended with domain-specific knowledge, offering unique opportunities in the natural sciences to accelerate our general understanding of the processes shaping our world. In this talk, I will try to demonstrate through three different projects how we can combine machine learning methods and process-based models to advance our understanding of living systems. I will first present a deep-learning based method to solve high dimensional non-local nonlinear PDEs, which can be used to simulate eco-evolutionary models of biological populations with many traits. I will then present a machine learning framework for inverse ecosystem modeling. The ML framework allows to estimate parameters of ecosystem models with complex dynamics, and perform eco-evolutionary model selection. Third, I will present a novel biodiversity model designed to attribute changes in biodiversity to global change. The model is based on a combination of general ecological principles, namely species-area relationship and niche theory, and machine learning. These three projects should provide a glimpse on how the combination of eco-evolutionary theory and machine learning can massively accelerate the underpinning of general principles at the ecosystem level, and provide reliable forecasts.
Date
14:00
Localisation
LIPhy, salle de conférence
- Share
- Share on Facebook
- Share on X
- Share on LinkedIn