- Share
- Share on Facebook
- Share on X
- Share on LinkedIn
Thesis defence
On December 16, 2024
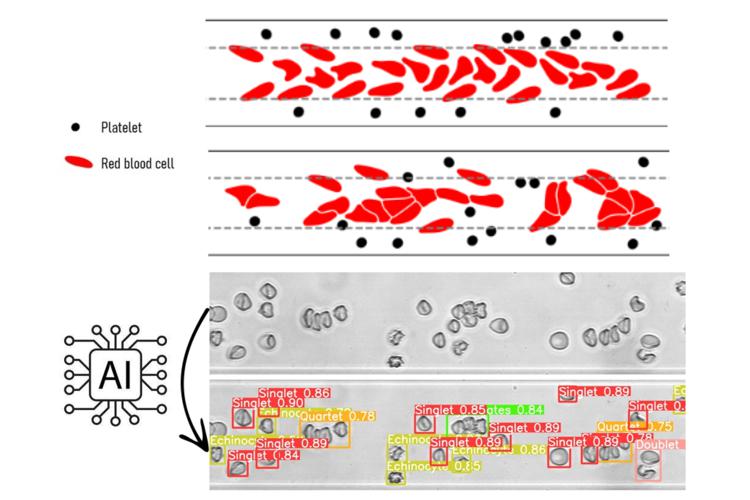
Mariam Dynar (ECCEL)
Proper blood perfusion in the microcirculation is essential for the delivery of oxygen and nutrients to tissues and organs. Since red blood cells (RBCs) are the major components of blood, the blood rheology is primarily controlled by RBCs. Under physiological conditions, and during their travel through blood vessels, RBCs undergos deformation, stack to each other (aggregate), and align to flow efficiently, ensuring the effective transport of oxygen and nutrients to tissues. However, in several diseases, like diabetes, RBC aggregates become stronger and more stable (the RBCs do not dissociate after forming an aggregate) due to the excess of fibrinogen (fibrinogen is the main protein responsible for RBCs aggregates formation, its normal concentration is 3.24 ± 1.39mg/ml), which may compromise blood flow and potentially lead to vascular occlusions. Moreover, due to the large number of RBCs and their interactions with other blood components, a simple change in RBC properties can influence the behavior of these components. For example, it is known that injuries in patients with diabetes take longer to heal compared to healthy individuals. Since platelets are the components responsible for thrombosis, and their margination toward the blood vessel wall is a key step in the thrombus formation process, we decided to study the effect of RBC aggregation on platelet margination, which has not been previously addressed in the existing literature.
This thesis focuses on RBC aggregation and its impact on platelet margination, as well as exploring the application of deep learning algorithms to RBCs aggregate analysis. Firstly, we numerically investigate the effect of adhesion energy between RBCs under Poiseuille flow on the RBCs aggregation. Initially, we focused on RBC doublets and found that, under certain conditions, they remain strongly attached even at high flow strengths. We then extended our study to the rheology of RBC suspensions under Poiseuille flow in both straight channels and bifurcations. This analysis was conducted using a combination of the lattice Boltzmann method and an immersed boundary method. Secondly, we investigated platelet margination in a straight channel with the presence of adhesion energy among RBCs. Our findings indicate that moderate adhesion energy, typical of normal physiological conditions, enhances the margination process. However, when the adhesion energy is sufficiently high, it results in a decrease in platelet margination. Finally, the manual detection and classification methods of RBCs aggregates require immense time, and it is possible that errors may be made during the classification and detection stage. We proposed a lightweight deep learning models called YOLO (You OnlyLook Once), that detect and classify the RBCs aggregates based on their sizes and also detect abnormal RBCs (like echinocyte). We collected our data by conducting microfluidic experiments using microfluidic devices with channels of different sizes and shapes.
Date
15:00
Localisation
LIPhy, salle de conférence
- Share
- Share on Facebook
- Share on X
- Share on LinkedIn