- Imprimer
- Partager
- Partager sur Facebook
- Share on X
- Partager sur LinkedIn
Séminaire
Le 19 décembre 2024
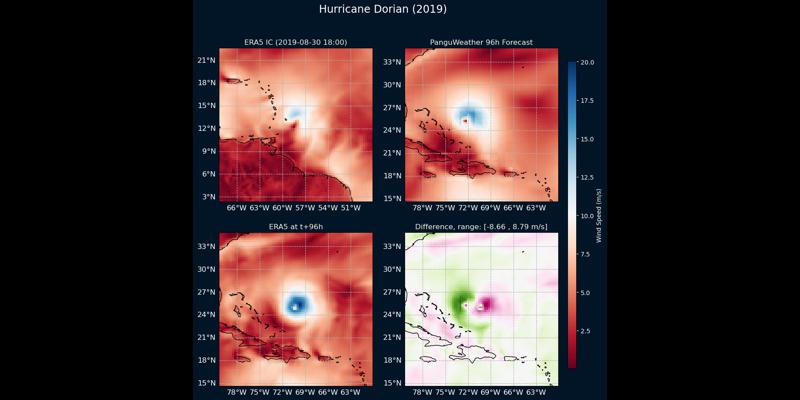
Milton Gomez (UNIL, Switzerland)
Bridging Machine Learning and Atmospheric Science: Advancing Weather Forecasts with Neural Models.
Milton Gomez (UNIL, Switzerland)
Numerical Weather Prediction (NWP) models, which integrate physical equations forward in time, are the traditional tools for simulating atmospheric processes and forecasting weather in modern meteorology. With recent advancements in deep learning architectures, Neural Weather Models (NeWMs) have emerged as competent NWP emulators with reported performances that compare favorably to the European Center for Medium-range Weather Forecast (ECMWF) models. However, they are commonly trained on meteorological reanalysis with limited spatial resolution (e.g., 0.25° horizontal grid spacing) and thus smooth out key features associated with Tropical Cyclones (e.g., wind gusts). To address this, we use our best global observational estimate of wind gusts and minimum sea level pressure to train models to post-process NeWM outputs and enable more accurate and reliable forecasts of TC intensity. In this presentation, we will go through a brief introduction to NWP and the advent of NeWMs, introduce a simple post-processing framework, and show the increase in prediction performance of naïve models through use of NeWM outputs.
Date
12:00
Localisation
LIPhy, salle de conférence
- Imprimer
- Partager
- Partager sur Facebook
- Share on X
- Partager sur LinkedIn