- Imprimer
- Partager
- Partager sur Facebook
- Share on X
- Partager sur LinkedIn
Séminaire
Le 3 juillet 2023
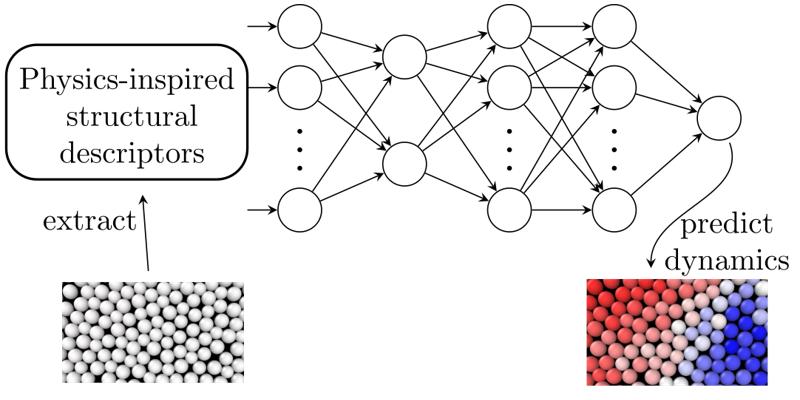
Gerhard Jung (Laboratoire Charles Coulomb, Montpellier Université, CNRS)
Understanding the precise nature of the glass transition is still an open problem despite many decades of research. Two of the most fundamental questions concern the connection between the disordered, microscopic structure and the emergent heterogeneous relaxation, as well as the efficient sampling of ultrastable disordered configurations. Both of these problems show surprising analogies to challenges in the field of machine learning: picture recognition and generative modeling.
In this talk I will give an introduction on how to use different machine learning techniques to tackle challenges in glass physics, and highlight potential applications in more general soft matter and biological systems. First, I will demonstrate how dense neural networks can be trained to predict correlations between disordered structures and emergent dynamical properties in densely supercooled liquids. These networks can then be analyzed to better understand dynamic heterogeneities at unprecedented low temperatures. Afterwards, I will present very recent results using unsupervised generative modeling to tackle the sampling problem. Using trained generative models it is possible to transform high temperature into low temperature equilibrium supercooled liquid configurations for efficient population annealing.
Date
14:00
Localisation
LIPhy, salle de conférence
- Imprimer
- Partager
- Partager sur Facebook
- Share on X
- Partager sur LinkedIn