- Imprimer
- Partager
- Partager sur Facebook
- Share on X
- Partager sur LinkedIn
Séminaire
Le 29 janvier 2024
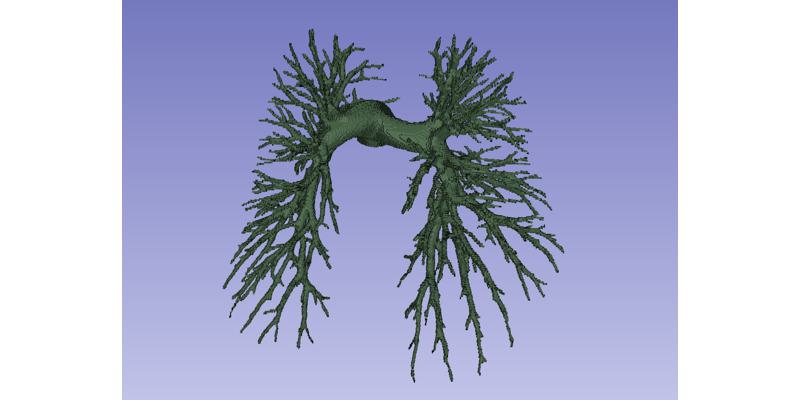
Odyssée Merveille (Creatis, INSA Lyon)
The segmentation of blood vessels in medical images is challenging as they are thin, tortuous and organized in networks.
Despite more than two decades of research, achieving a complete and connected vascular network segmentation remains a challenge, in particular when dealing with 3D images. Yet, a geometrically accurate segmentation is critical for clinical applications such as cardiovascular disease computer-aided diagnosis and surgical planning.
End-to-end deep learning approaches have been extensively studied for medical image segmentation. However, state-of-the-art approaches yield sub-optimal results when applied to vascular segmentation. The segmentations tend to be disconnected, leading to poor results in downstream tasks such as blood flow simulations. Moreover, these learned models are not robust to domain shift, which drastically reduce their performances when applied to datasets without annotations.
In this talk, I will present several strategies that we proposed to improve the segmentation of vascular network with deep learning approach. First, I will discuss two approaches designed to enforce the connectivity of vascular networks. Then, I will present domain adaptation strategies that can be used to segment vascular image datasets from which no annotation is available.
Contact: Aurélien Gourrier
Date
14:00
Localisation
LIPhy, salle de conférence
- Imprimer
- Partager
- Partager sur Facebook
- Share on X
- Partager sur LinkedIn