- Share
- Share on Facebook
- Share on X
- Share on LinkedIn
Development of statistical tools for the analysis of microbial growth and gene expression data
Aline Marguet, Eugenio Cinquemani
Non-permanent: Emrys Reginato (PhD student), Claudia Fonte Sanchez (postodoctoral researcher)
Dynamical growth experiments on microbial cultures generate a wealth of measurement time series such as absorbance and fluorescent reporter evolution profiles (microplate and bioreactor experiments), cell elongation and divisions (videomicroscopy), and sample statistics of single-cell measurements (flow cytometry).
These data are rich but also typically noisy and incomplete. Most often, it only provides an indirect monitoring of the cellular processes of interest (growth rate, gene activation and regulatory dynamics, etc.). The variety of existing monitoring technologies and their constant development motivate us to develop dedicated methods for data treatment and information extraction.
Leveraging estimation theory, machine learning approaches and mathematical modelling, we develop methods for statistical data processing (noise removal, smoothing, parameter fitting, ...) and the reconstruction of hidden cellular dynamics from time-course data. Typical examples include algorithms for estimation of gene expression and resource allocation dynamics from time-course fluorescent reporter data, identification of dynamical regulatory interactions among genes, reconstruction of intrinsic and extrinsic noise sources.
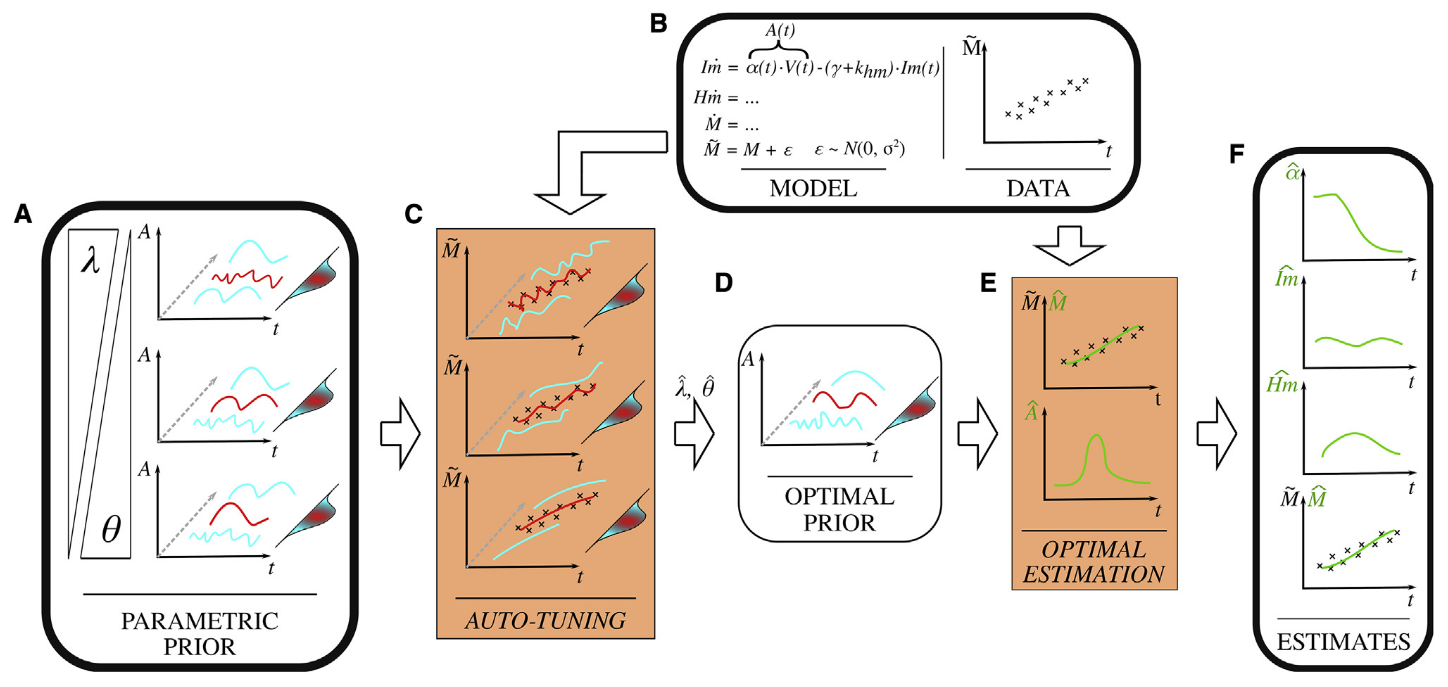
- Share
- Share on Facebook
- Share on X
- Share on LinkedIn